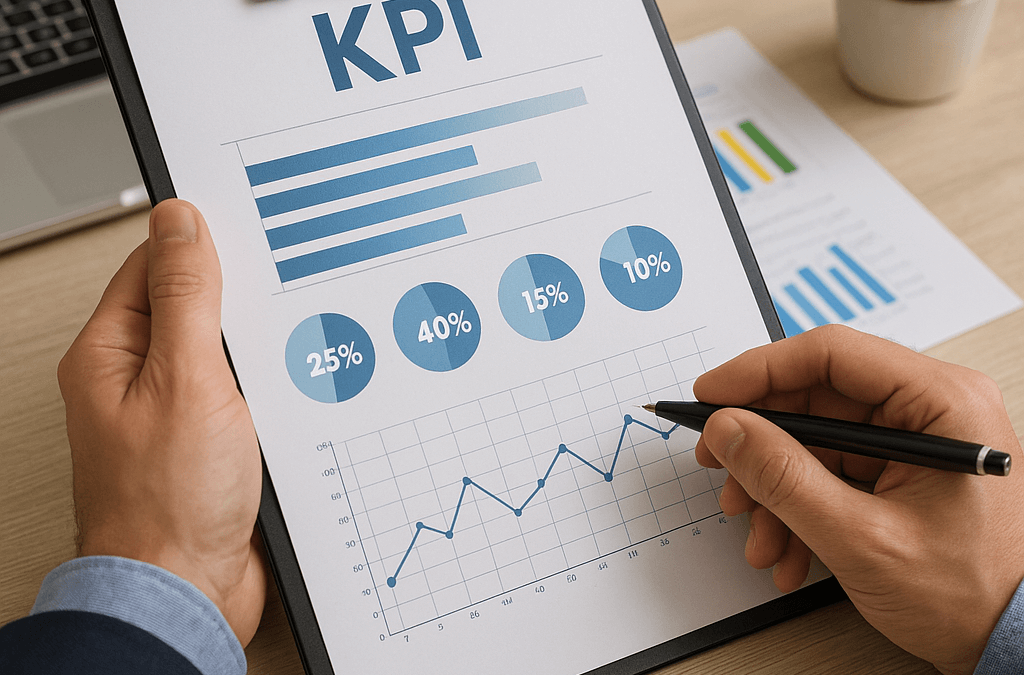
Are Your KPIs Actually KPIs?
In nearly every organization, Key Performance Indicators (KPIs) are a staple of performance tracking and strategy alignment. But how often do we stop to question whether our KPIs are truly key, or even indicators of performance? Was there an analysis done to evaluate the efficacy of these KPIs? At what value, for a given KPI, do we need to take action or intervene? From what we’ve seen, that answer to these questions often leaves a lot to be desired.
Too often, businesses select KPIs that are not actually impactful but provide a false sense of security because data is being analyzed and tracked. We see organizations track metrics like total page views, hours worked, or number of meetings scheduled that tell us little about actual business outcomes. These metrics are often shown on a dashboard and are not tied to any specific decision or intervention.
Effective KPIs should be tied directly to a decision. If a KPI doesn’t drive an action or inform a choice, it’s just adding to the noise. For example, tracking average customer response time is only valuable if it helps improve satisfaction or retention. For example, at what level of customer response time does it become justifiable to intervene to protect retention? If it’s measured but ignored, it’s not a KPI, it’s background data. While that may be useful or worth tracking, if it isn’t impacting decisions or flagging areas to intervene, it’s not a KPI.
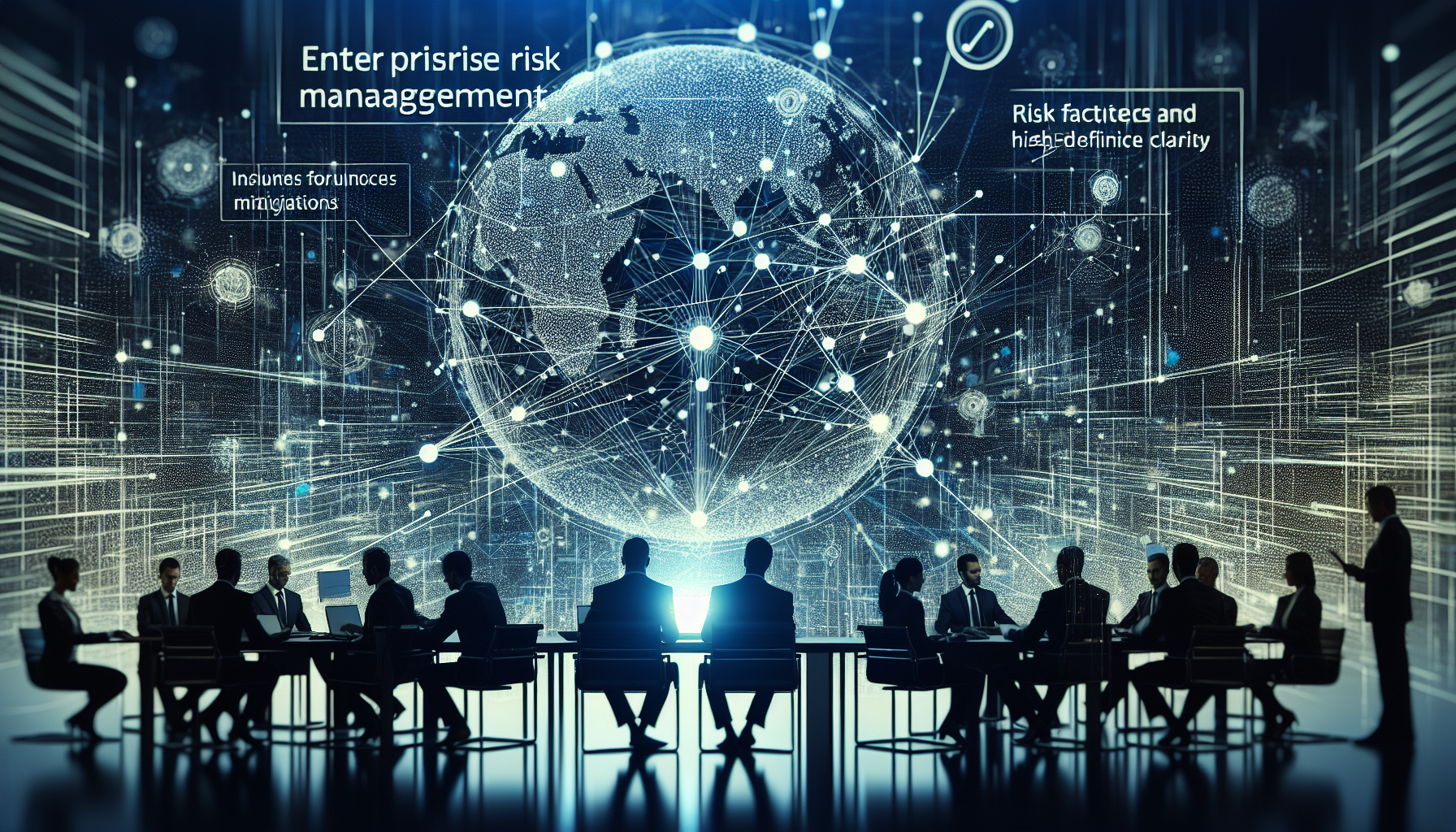
At HDR we have observed a phenomenon called the Measurement Inversion (see Figure 1) in almost every single industry we’ve worked in. Organizations are often measuring and tracking data (at great expense) that has no actual influence on a decision. When we run information value calculations in decision models, we routinely see little-to-no value in the metrics that are being tracked and massive value for other metrics in the model (metrics that could actually influence a decision one way or the other).
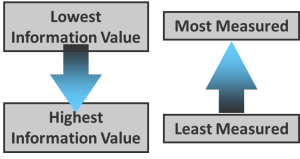
Figure 1: Depiction of the Measurement Inversion
At the end of the day, KPIs are not only about data, they’re about decisions. The most valuable metrics illuminate what matters, show what’s working, and prompt better actions. If your current KPIs don’t do that, it’s time to rethink what you’re measuring and how you’re measuring it.