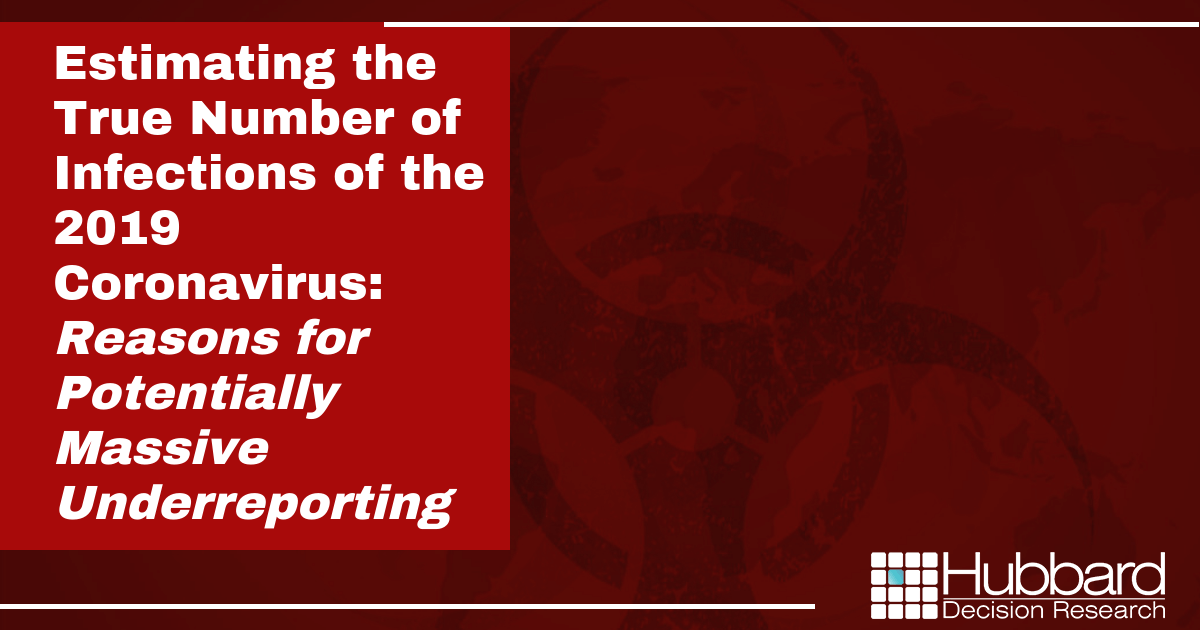
There is good reason to believe that the number of the 2019 novel coronavirus- or 2019-nCoV – infections worldwide may be much greater than what has been reported so far. As of Tuesday, February 3, 2020, at 8:00am CST (UTC-6) the Johns Hopkins coronavirus tracking map shows there are 17,489 confirmed inflections. Of course, even in the best reporting systems, augmented with the fastest diagnoses, any number of reported infections would almost certainly be too low. However, our analysis points to a number of infections that may be in the hundreds of thousands, or possibly over one million – far above the latest official numbers.
Many informative analyses already exist on this outbreak, and it was relatively easy to build a model that accurately predicts the next few days of deaths and reported infections (Figure 1).
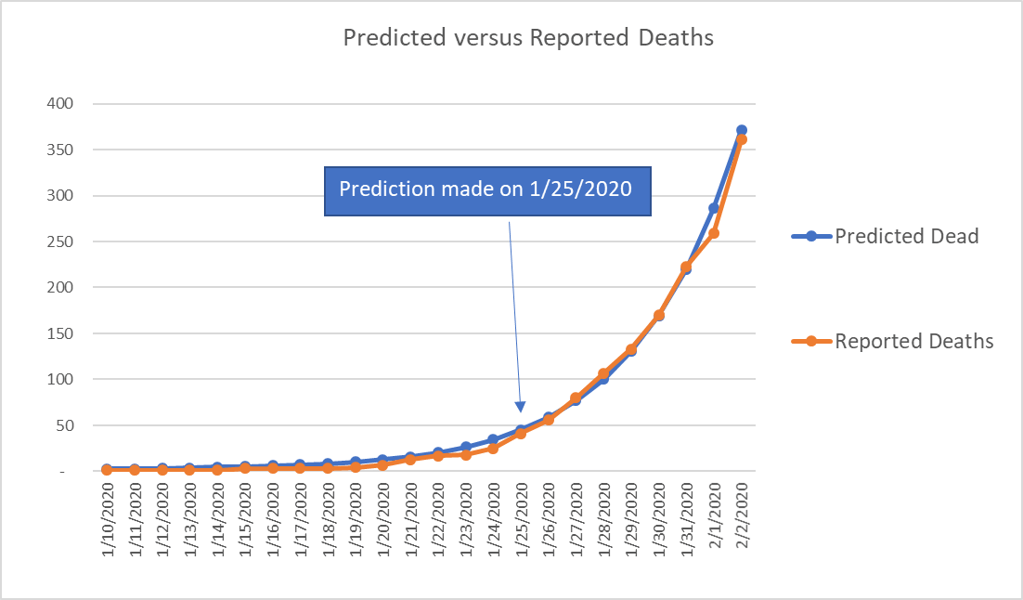
Figure 1: Prediction made on 1/25/2020 using a naïve exponential growth (daily) of 0.26 and a probability distribution function for time to death based off initial data. (http://www.nhc.gov.cn/yjb/s3578/202001/5d19a4f6d3154b9fae328918ed2e3c8a.shtml).
There is much more uncertainty regarding the total number of cases and infections. This number is crucial because it can inform the international community in terms of expected cases. The number of expected cases then informs decision-making and resource allocation from governments and non-governmental organizations (NGOs) to combat the spread. Too much uncertainty in either direction – overestimating versus underestimating – can result in efforts that are inefficient at best and ineffective or even harmful at worst.
While articles already exist that put the number of total cases at 75,000, we believe there is still a crucial distinction that hasn’t been incorporated: the incubation time and exponential growth of the virus, which can make the infections an order of magnitude higher than the symptomatic cases.
If the calculations in this article are correct, then there are already dozens of cases of 2019-nCoV in the United States, many of which will go undetected.
We support these assertions using two different methods of calculation, both of which depend, in part, on the fact that the average time to death from infection is long (25 days or greater on average, based on initial data). These calculations also depend on the observations that the average time from becoming infected to going to the hospital is 10-15 days; that only 20% of those infected ever require hospitalization; and that average time from hospitalization to death is relatively long (12 to 18 days).
Based on these calculations, we encourage world citizens and governments to all act now to prepare for a world-wide pandemic.
We begin by stating the summary of concerns:
- 2019-nCoV is not like the seasonal flu – it is more contagious, has a longer incubation period, has a much higher rate of serious complications (ICU bed requirement), and is deadlier, with a fatality rate that is five to 30 times higher.
- Curious patterns in the data indicate the possibility of massive underreporting.
a. This could partly due to the characteristics of the disease itself – a long incubation period and significant period of time from initial
symptoms to the development of more serious symptoms.
b. It could also be due in part to human systems for detecting the disease. Even assuming competence and best intentions, there are factors that will contribute to under-reporting in the early parts of an epidemic and during the period of exponential growth.
We remain impartial regarding whether China is intentionally misrepresenting data. Regardless, social media videos out of China on 1/23/2020 appear to indicate a gap between what was reported and what was actually occurring on the ground in the Wuhan province. Keep in mind that at the time China had reported only 21 deaths, while Wuhan was treating coronavirus in 20+ hospitals. While the baseline for hospitals in China may be more chaotic then Europe or the US, the videos do not appear to be consistent with something less impactful than the seasonal flu. Here is a scene from one of those Wuhan hospitals on 1/23/2020.
The Importance of Symptomatic Vs. Infected
As we mentioned, organizations across the globe are rushing to provide analysis and understanding. What we haven’t seen yet in any analysis, however, is a sobering realization: that the length of time from infection to death implies a number of infections that is two orders of magnitude higher than the current reports.
The lengthy incubation period represents a lag between the time someone becomes infected by the virus and when they begin to show symptoms. The incubation period for the common cold is anywhere from 24 to 72 hours; for the garden-variety flu, it’s two days on average. The incubation period for the Spanish flu, the deadliest pandemic in the history of the world after the Black Death, had an incubation period of two to seven days.
2019-nCoV, by contrast, has an incubation period that lasts an average of five to 10 days.
Incubation periods matter because the longer infected persons go without showing symptoms, the longer they go without seeking care and the more people they expose to the pathogens they carry inside of them. Calculating the number of people who have shown symptoms of 2019-nCoV, then, carries the risk of grossly undercounting the number of people who are actually infected.
A compelling picture of this issue is illustrated by comparing the number of infections and the number of symptomatic cases (Figures 2-4).
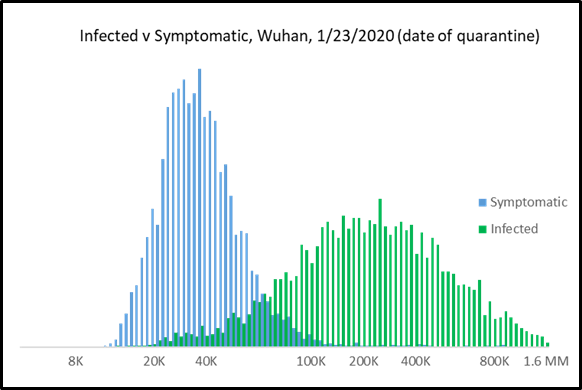
Figure 2: Monte Carlo Simulation results illustrating difference between infected and symptomatic cases (log scale).
Below we include individual histograms for both infections and symptomatic cases that illustrate the difference between reported cases, calculations based on current international symptomatic cases, and our calculations based on a Monte Carlo model that incorporates the incubation period. Note the scale on infections (Figure 4) is 10-15 times larger than the scale on symptomatic cases (Figure 3).
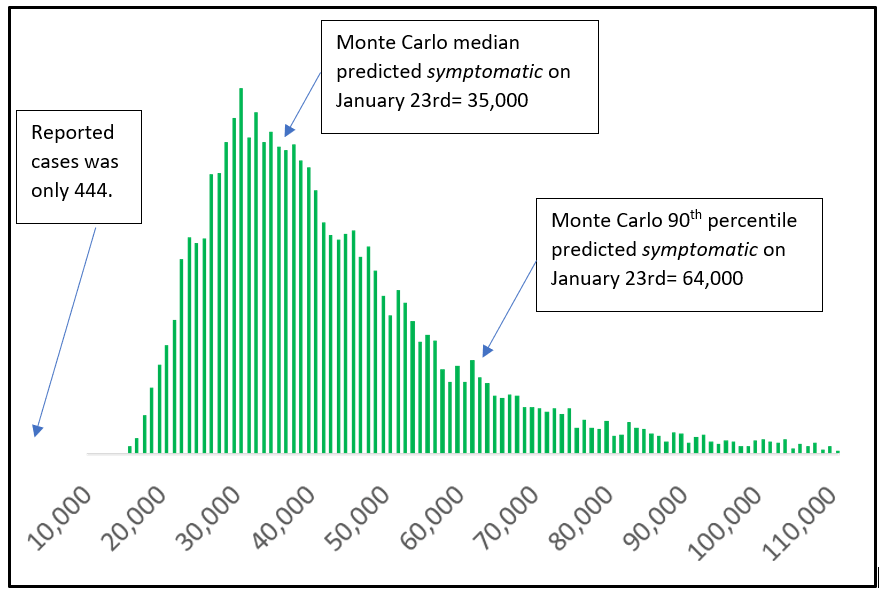
Figure 3: Symptomatic Cases of 2019-nCoV in Wuhan on Jan 23rd (histogram of 10,000 trials of a Monte Carlo model).
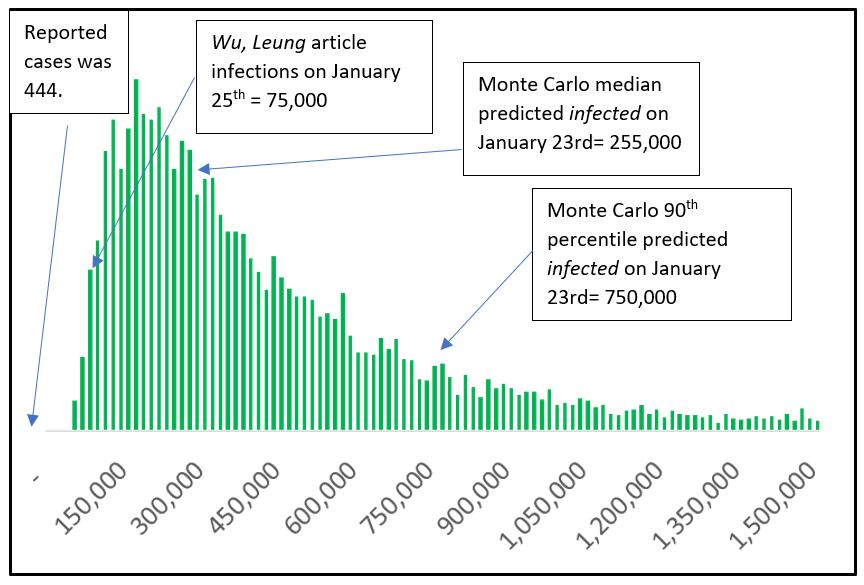
Figure 4: Infections of 2019-nCoV in Wuhan on Jan 23rd (histogram of 10,000 trials of a Monte Carlo model).
Even Thursday’s Lancet paper, which is backing out 75,000 Wuhan infections as of 1/25/2020 is low for the same reason – they are using international “infections” as their starting point and observing it is an unrealistic number given a mere 3,300 daily international passengers out of Wuhan before the quarantine. But the results are likely low because of the nature of growth and long incubation period of this virus – they can only count the “symptomatic” international cases – not the infected international cases.
How Lagging Indicators Obscure Reality
Sometimes, our understanding of the actual magnitude of an event, like a pandemic, is limited by the fact that even in the best of circumstances, data often lags behind the pace of reality.
Based on the published case history of the first 17 deaths occurring from 2019-nCoV, the average time from infection to death is 22-35 days (1-14 days for incubation, five days from onset of symptoms to hospitalization, and 12-18 days from hospitalization to death). In addition, only 20% of those infected ever require hospitalization.
We also have the epidemiological reality that time to death is always underrepresented during the exponential growth phase of an epidemic because the people who take a long time to die haven’t died yet.
The implications of this long time from infection to death makes it difficult for the public to comprehend, during the early stages, the stunning nature of the implied exponential growth in cases.
Put another way, the situation could very well be worse than we believe because we expect the numbers we receive in the news to be closer to the truth than what they really are: a snapshot of the past that grows older and more obsolete at a dizzying pace.
To be conservative, let us assume that the average time to death from infection is 25 days (the Monte Carlo simulations with the best fit to deaths is 29 days from infection to death). This implies that, given a 2.5% fatality rate, and that 362 people have died, there were 14,480 cases 25 days ago. Stated otherwise, given that it takes 25 days to die from infection, 14,480 were infected as of 1/9/2020! (At the time, the official number of confirmed cases was just 41.)
Combine this with a fearsome exponential growth from 1/9/2020 until the quarantine on 1/23/2020 and one starts to get the picture.
Shedding Light on Exponential Growth Rates with Probabilistic Models: How Many People Are Really Infected?
We still can’t make a definitive estimate of exponential growth, in part, because it changes over time as behaviors change. However, the beauty of a probabilistic model, one using initially wide ranges for variables, is that we can quickly discern certain values of variables that are unrealistic. In other words, the model can rapidly narrow the wide amount of uncertainty we have about a given variable (i.e. infection rates).
Using this method, we have concluded that the unrestrained (i.e., before quarantine) log growth rate of 2019-nCoV is at least 0.26 and as high as 0.31. This implies a doubling time in the initial stages of the outbreak of between 2.3 and 2.7 days – far less than other published estimates. A shorter doubling time represents the virus spreading faster among a given population. When coupled with the other observations the international community has gathered about the virus, the models give us a range of likely outcomes that reduce the uncertainty to as much as possible given what we know now.
The results are disquieting. Our best estimates for current infections are 800,000 in Hubei/Wuhan and 337,000 in the rest of China (Table 1).
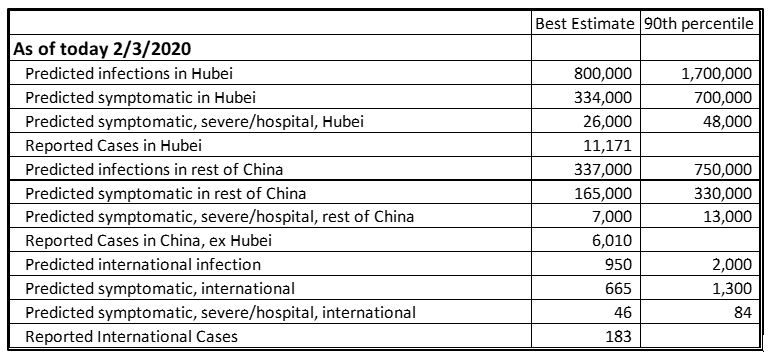
Table 1: Monte Carlo predictions for current levels of 2019-nCoV in Hubei, China ex Hubei, and International.
How Could This Be?
Skeptics at this point will say, “How could 1,000,000 people in Wuhan be sick? That would imply that every 8th person was sick and that would be all over the media!”
First, there has been very little reporting out of Wuhan in the last week. Governments at all levels have moved to restrict access into and out of the quarantine zone, leaving first-hand news via uncontrolled sources in scant supply.
Second, there is a big difference between 1,000,000 infected and 1,000,000 sick. Because the incubation period is so long, even if 1,000,000 were infected, only a few hundred thousand might be symptomatic.
Skeptics might still say, “How could even 300,000 be sick when the official number is only 11,000?” Recall that the average time from becoming symptomatic to going to the hospital is five days, and that only 20% ever require hospitalization. That means only 60,000 of those 300,000 will require hospitalization. Add in the average lag between being symptomatic and hospitalization, and physical limitations at hospitals, and 11,000 is a very reasonable number of reported cases.
Therefore, it is entirely believable that 1,000,000 people are infected with 2019-nCoV as of 2/3/2020, and with 500,000 infections realized before the time the quarantine was in place on 1/23/2020.
If this is true, then at least 200 infected people flew to international destinations before the quarantine, and 75,000 infected people traveled to domestic Chinese destinations before the quarantine. Given the exponential rate of growth discussed previously, it is possible – perhaps likely – that the original Wuhan quarantine was too little, too late to keep the pandemic from spreading.
Implications for Mainland China
The bad news is that given there are likely hundreds of thousands of infections in China, it is unlikely that China will be “open for business” in the next month – possibly even the next several months. Undoubtedly, the unprecedented quarantine and public health measures China has taken will have reduced the log growth rate of new cases. However, because of the long incubation time and the uncertainty around reporting, we will have wide error bars around our estimate of the new rate of growth for several weeks.
Implications for Other Countries
Given how contagious the disease is, and the continued free flow of international travel from other Chinese ports up through 2/1/2010, an international pandemic likely at this point.
However, there is reason to be encouraged by the international response thus far. The numbers of reported and predicted infections are relatively low, and many people with the virus have largely self-quarantined, which helps tremendously.
The biggest issue is that our sample of infected people is going to be skewed toward the responsible people. Responsible actors will appear in reports; less responsible actors may not. People who have visited China in January and don’t self-quarantine, or who display very moderate symptoms, could slip through the cracks. There is already evidence that infected people with few symptoms can infect clusters of people. The possibility for an epidemic in another country comes if these clusters aren’t immediately caught.
How might this happen? Here is an actual story that has already been reported. An older couple from Wuhan visited their daughter in Shanghai some time during the week before 1/15/2020. They were infected with 2019-nCoV; the daughter soon caught it as well. The daughter then flew to Germany on the 15th and spent a week with colleagues before returning to Shanghai on 1/22/2020. She only became symptomatic after leaving Germany, but had infected several Germans before doing so.
What Do I Do?
Hope is not a good strategy to mitigate risk. While positive interpretations of the data exist, counting on that to be reality sets us up to repeat the initial mistakes in early January to contain and mitigate the virus.
Most humans don’t like to think about things like this because cold, uncompromising reality is uncomfortable, forcing us to decide to either change our habits or face the possibility of disease or death. Nevertheless, making rational decisions to protect yourself based on a sound statistical and logical analysis has helped many millions of lives in the course of human history.
Our recommendation is that we directly address the issue that the current and eventual spread and magnitude of the outbreak could be worse than we believe or imagine, bearing in mind that it is possible we may only have a few days before outbreaks start popping up in the U.S. and possibly just weeks before a general epidemic begins stateside.
Part of the resistance to thinking about pandemics is that it is easy (but incorrect) to assume that we are powerless to affect the outcome. Here are three easy things we can do on a personal level:
- Wash our hands. This isn’t as easy as it sounds – it means creating a new habit where we wash or disinfect our hands whenever we’ve contacted a public surface (grocery cart, bathroom door handle, etc.). Disinfecting helps. Washing and scrubbing with soap for 15 seconds is the formal protocol.
- Some published research shows that elderberry syrup may reduce the severity of other types of flu viruses and reduce the probability of transmission.
- If there is a cluster or outbreak in your city, minimize or avoid public gatherings and wear protective facemasks in public. This is probably the most difficult one because it comes at a high social cost some people aren’t willing to pay.
Preparations for Your Business
If you’d like help preparing your business for the possibilities, there are certain practices you can adopt now that will give you an advantage regardless of outcome (such as reviewing your capabilities for remote work). Also, if you’d like to use or test scenarios on the Monte Carlo model we created, please contact us. One of the advantages of using a probabilistic model is that you can update the uncertainty in real time and always know what contingencies you need to put in place for the most likely scenarios.
Matt Millar is a senior quantitative analyst with Hubbard Decision Research. Contact Matt for more information on this article or any of the methods discussed wherein.